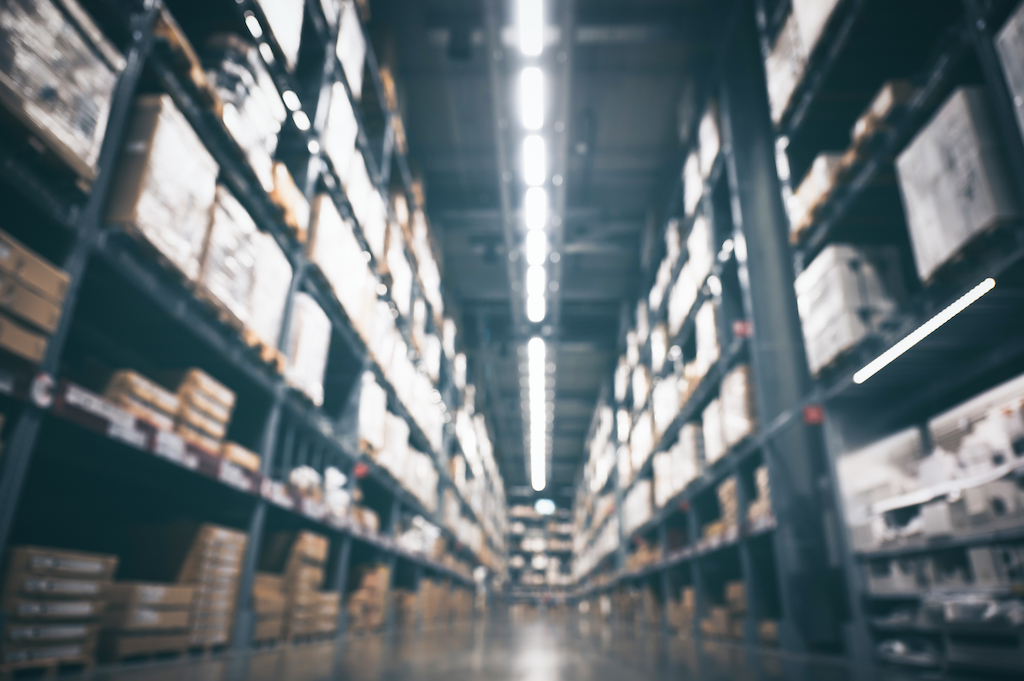
Leveraging AI: Unlocking the Power of Inventory Management
Introduction:
In today’s highly competitive market, organizations cannot afford to rely on outdated manual inventory management methods. These traditional approaches are not only time-consuming but also prone to errors due to human limitations. Additionally, they struggle to tap into the wealth of data available that could provide valuable insights for optimizing inventory control. This is where the integration of Artificial Intelligence (AI) into inventory management processes has proven to be a game-changer.
AI empowers businesses with sophisticated algorithms and machine learning capabilities, enabling them to make data-driven decisions, automate processes, and achieve higher levels of efficiency and accuracy. By leveraging the power of AI in inventory management, organizations can streamline supply chain operations, reduce costs, enhance customer satisfaction, and stay ahead of the competition.
Let’s explore some of the key benefits that AI can bring to inventory management:
1. Demand Forecasting:
Demand forecasting is a critical aspect of effective inventory management. Accurate predictions of future demand allow organizations to optimize inventory levels, minimize stock-outs, and reduce excess inventory. This is where AI-powered systems excel, utilizing advanced algorithms to analyze a vast array of data sources and generate highly accurate demand forecasts.
One notable example of AI-driven demand forecasting is Amazon. With its tremendous scale and vast product assortment, Amazon relies heavily on AI and machine learning to manage its inventory. By analyzing historical sales data, customer browsing behavior, purchase patterns, and even external factors like weather patterns and social media sentiments, Amazon’s AI-powered system can predict future demand with remarkable accuracy.
For instance, during holiday seasons or special promotions, Amazon can forecast increased demand for specific products based on historical data and customer browsing patterns. By leveraging AI in demand forecasting, Amazon adjusts its inventory levels accordingly, ensuring adequate stock availability to meet customer demand while minimizing excess inventory that ties up capital and increases carrying costs.
The benefits of AI-driven demand forecasting extend beyond just maintaining optimal inventory levels. Companies can use these insights to make more informed decisions regarding production scheduling, purchasing, and even pricing strategies. For instance, if the AI system predicts a surge in demand for a particular product, the company can adjust production schedules or negotiate favorable terms with suppliers in preparation for the anticipated increase in demand.
Additionally, AI-powered demand forecasting can help organizations identify emerging trends and adapt their product offerings accordingly. By analyzing social media sentiments, customer reviews, and market trends, companies can spot changing consumer preferences and adjust their inventory mix. This proactive approach enables organizations to stay ahead of the curve and meet customer expectations, boosting customer satisfaction and loyalty.
However, it’s important to note that AI is not infallible, and demand forecasting may still face uncertainties and unexpected fluctuations. External factors like political events, natural disasters, or sudden shifts in consumer behavior can influence demand patterns beyond what historical or social media data can predict. Therefore, companies must not solely rely on AI-generated forecasts but should also incorporate human judgment and real-time market intelligence to make informed decisions.
By harnessing the power of AI in demand forecasting, organizations can optimize their inventory management practices and achieve several benefits. These include reducing stock-outs, minimizing excess inventory and associated costs, enabling proactive decision-making, and enhancing customer satisfaction. The remarkable efficiency and order fulfillment rate demonstrated by Amazon’s AI-driven inventory management system serve as a perfect example of how effective demand forecast can significantly impact business performance.
2. Process Automation:
Process automation is a key area where AI can drastically improve inventory management. By leveraging AI technologies, organizations can automate repetitive and time-consuming tasks involved in inventory tracking, supply chain optimization, and order fulfillment. This not only reduces the risk of human error but also enables employees to focus on more strategic activities, ultimately leading to improved efficiency and reduced operational costs.
One prominent example of AI-driven process automation in inventory management is Alibaba, one of the world’s largest e-commerce companies. Alibaba utilizes AI extensively in its inventory management system to automate various tasks, including real-time inventory tracking. As sales occur, the AI system automatically updates inventory levels, ensuring accurate and up-to-date information.
With real-time inventory tracking, Alibaba can streamline its purchasing processes by having clear visibility of stock levels and automatically placing orders to restock when inventory reaches a certain threshold. This proactive approach to inventory management minimizes the risk of stockouts and improves order fulfillment rates. Additionally, the system allows Alibaba to provide customers with accurate and reliable order fulfillment estimates, enhancing the overall customer experience.
Furthermore, AI-powered process automation in inventory management extends beyond inventory tracking. By analyzing historical data, supplier performance, lead times, and market trends, the AI system can optimize supply chain logistics, helping organizations minimize costs and improve operational efficiency.
For example, Alibaba’s AI system can intelligently forecast demand based on customer behavior and market trends. This information allows them to optimize their supply chain by identifying the most cost-effective transportation routes, reducing lead times, and ensuring the right products are available at the right locations. By automating these processes, Alibaba can improve its operational efficiency, deliver products faster, and ultimately provide better customer service.
However, it’s important to consider the limitations of process automation through AI. While AI systems can perform tasks with speed and precision, they may struggle to adapt to unforeseen circumstances or handle complex scenarios that require human judgment. Human oversight and intervention remain crucial to ensure that AI-generated outputs align with business goals and address unique situations.
AI-driven process automation in inventory management offers significant benefits to organizations. By automating tasks such as inventory tracking, supply chain optimization, and order fulfillment, businesses can reduce errors, improve efficiency, and lower operational costs. Alibaba’s AI-powered inventory management system serves as a prime example, demonstrating how automation can enable real-time inventory tracking, streamline purchasing processes, and enhance customer satisfaction. As organizations continue to leverage AI technologies, they can unlock the full potential of process automation in inventory management to gain a competitive advantage in the market.
3. Optimal Inventory Control:
Optimal inventory control is crucial for organizations to minimize carrying costs and improve overall profitability. AI algorithms play a vital role in analyzing enormous volumes of data to identify the ideal inventory levels at various stages of the supply chain. By leveraging AI in inventory control, companies can reduce excess inventory while ensuring adequate stock availability, leading to optimized cash flow and increased operational efficiency.
One of the prime examples of AI-enabled optimal inventory control is Walmart. As one of the largest retail chains globally, Walmart utilizes AI extensively in its inventory management system. The AI system continuously monitors sales patterns, market trends, and customer behaviors to make data-driven decisions regarding pricing, promotions, and replenishment.
Through AI analysis, Walmart can accurately predict demand for products at different locations and adjust their inventory levels accordingly. This ensures that stores have sufficient stock to meet customer demand while minimizing overstocking. By achieving the optimal balance between inventory levels and customer demand, Walmart reduces stockouts, improves customer satisfaction, and avoids the costs associated with excess inventory.
Walmart’s AI-enabled system also aids in dynamic pricing and promotions. By analyzing data on customer preferences, competitor pricing, and market demand, the AI system can recommend optimal pricing strategies for different products in different locations. This allows Walmart to maximize revenue and profitability while remaining competitive in the market.
Furthermore, AI algorithms contribute to efficient replenishment processes. The system can forecast when stock levels will reach reorder points and automatically generate purchase orders or trigger alerts for manual intervention. This proactive approach aids in avoiding stockouts and ensures a smooth flow of products through the supply chain.
However, it is important to note that AI algorithms for optimal inventory control require accurate and relevant data inputs. Organizations should continuously monitor the quality and integrity of data sources to ensure accurate demand forecasting and inventory optimization.
AI-powered optimal inventory control provides significant benefits to organizations by minimizing excess inventory, reducing carrying costs, and improving cash flow. Walmart’s AI-enabled inventory management system serves as an excellent illustration of the advantages gained through accurate demand forecasting, dynamic pricing, and efficient replenishment processes. By leveraging AI in inventory control, companies can achieve operational excellence, increase customer satisfaction, and drive overall profitability.
Risks and Challenges:
While AI has the potential to significantly improve inventory management, its implementation comes with inherent risks and challenges that organizations need to be aware of and mitigate effectively. It is important to recognize and address these risks to ensure successful AI integration and minimize potential negative impacts. Let’s delve into the main risks and challenges associated with AI in inventory management:
1. Data Quality and Integration:
Data quality and integration are critical factors for the successful implementation of AI in inventory management. High-quality data ensures that AI algorithms generate accurate and reliable insights, allowing organizations to make informed decisions regarding inventory optimization and supply chain management. Here’s an in-depth exploration of data quality and integration in the context of AI for inventory management.
- Data Quality Assurance:
Organizations must establish robust data quality control processes to ensure the accuracy, completeness, and integrity of the data used for AI analysis. Some key steps to ensure data quality include:- Data Cleansing: Regularly audit and clean the data to eliminate errors, duplicates, and inconsistent values. This process helps maintain data accuracy and integrity, reducing the risk of faulty AI outputs.
- Data Validation: Implement processes to validate data accuracy and completeness at the point of entry and during integration. By validating data against predefined rules and standards, organizations can identify and rectify data issues promptly.
- Data Governance: Define and enforce data governance policies that outline data quality standards, data ownership, and maintenance responsibilities. This ensures accountability for data accuracy and promotes a culture of data-driven decision-making.
- Data Documentation: Properly document data sources, transformations, and cleaning processes. Transparent documentation enhances the traceability and reliability of data, enabling better understanding and auditing of AI models.
- Data Integration:
Data integration is crucial for AI systems to access and consolidate data from various sources within the organization. This integration allows for a unified and comprehensive view of inventory across the supply chain. Some considerations for effective data integration include:- Unified Data Architecture: Create a standardized data architecture that facilitates the integration of data from disparate sources, such as sales systems, supplier databases, inventory management platforms, and market trend data. Well-designed data integration frameworks streamline data access and facilitate data sharing across systems.
- Data Mapping and Transformation: Employ data mapping and transformation techniques to ensure seamless integration of data from various systems. This involves aligning data fields and formats to ensure consistency and compatibility between different datasets.
- Real-time Data Integration: Implement real-time data integration processes to enable timely access to data from different sources. This allows organizations to leverage up-to-date information for accurate demand forecasting, inventory tracking, and replenishment decisions.
- Advanced Analytics: Explore advanced analytics techniques, such as data lakes or data warehouses, to store and analyze large volumes of integrated data. These technologies provide a scalable infrastructure for AI algorithms to process and generate insights from diverse datasets.
By prioritizing data quality assurance and effective data integration, organizations can harness the full potential of AI in inventory management. Accurate and integrated data provides a solid foundation for AI algorithms, enabling enhanced demand forecasting, optimized inventory levels, improved supply chain efficiency, and ultimately, better business outcomes.
2. Implementation Costs:
Implementing AI systems for inventory management involves upfront costs related to infrastructure, software, and skilled personnel. These costs can present challenges, particularly for small and medium-sized businesses. However, with careful planning and a thorough cost-benefit analysis, organizations can justify the investment in AI and identify key areas where it can have the most significant impact. Let’s explore these aspects in more detail:
Infrastructure Costs:
AI implementation typically requires robust computational resources to handle the processing and analysis of large datasets. This may involve investing in servers, data storage facilities, cloud computing services, and networking infrastructure. The costs associated with acquiring and maintaining these infrastructure components should be considered during the planning stage.
Cloud-based solutions are often an attractive option for organizations looking to implement AI systems with reduced upfront infrastructure costs. Cloud providers offer scalable resources, allowing businesses to pay for what they use, reducing the need for substantial initial investments.
Software Costs:
AI software is a crucial element of implementing AI systems. It includes tools for data analysis, machine learning models, and AI algorithms. The costs may involve licensing fees, subscription fees for cloud-based AI platforms, or custom development costs if bespoke solutions are required.
When evaluating AI software options, organizations should consider factors such as features, scalability, ease of integration with existing systems, vendor support, and long-term sustainability.
Skilled Personnel:
Implementing and maintaining AI systems require skilled personnel, including data scientists, AI engineers, and domain experts. Hiring or training personnel with AI expertise can be costly, especially considering the demand and scarcity of AI talent in the job market.
Organizations have options for building AI capabilities in their teams. They can hire experienced professionals, partner with AI consultancy firms, or provide training and development programs to upskill existing employees. Careful consideration should be given to aligning AI talent requirements with the organization’s overall AI strategy and long-term goals.
Cost-Benefit Analysis:
Conducting a thorough cost-benefit analysis is crucial to assess the potential return on investment (ROI) of AI implementation in inventory management. This analysis should include factors such as:
- Cost savings from optimized inventory levels, reduced stockouts, and improved supply chain efficiency.
- Potential revenue growth resulting from enhanced demand forecasting, higher customer satisfaction, and improved product availability.
- Operational efficiency gains through process automation, reducing manual labor costs and reallocating employee time to more strategic tasks.
- Competitive advantages gained through improved decision-making and agility in responding to market dynamics.
By quantifying the benefits and comparing them to the implementation costs, organizations can make informed decisions on the viability and prioritization of AI projects in inventory management.
It is important to note that AI implementation costs can vary depending on the scale and complexity of the organization’s operations. Small and medium-sized businesses may focus on starting with smaller, targeted AI projects that offer immediate benefits and allow for iterative growth with the organization’s resources and capabilities.
Overall, by conducting a comprehensive cost-benefit analysis, organizations can assess the affordability and potential impact of implementing AI systems for inventory management. This analysis helps in justifying the investment, identifying areas to prioritize, and ensuring the best use of resources to achieve significant improvements in inventory optimization, supply chain efficiency, and overall business performance.
3. Overreliance on AI:
While AI algorithms provide valuable insights and automate various aspects of inventory management, organizations must be cautious of overreliance on AI without incorporating human judgment and expertise. While AI systems excel in analyzing large datasets and making accurate predictions based on historical patterns, they may not fully account for contextual nuances and unexpected events that can impact inventory needs. It is critical to strike a balance between AI capabilities and human oversight to ensure effective decision-making. Here are some key considerations for managing the risk of overreliance on AI in inventory management:
- Contextual Understanding: Human expertise and domain knowledge are essential for contextualizing AI outputs. While AI algorithms excel in analyzing data, humans bring a nuanced understanding of the market, customer behavior, and subtle factors that may not be captured within the datasets. By combining AI-generated insights with human judgment, organizations can make more robust decisions regarding inventory optimization and supply chain management.
- Validation and Verification: Organizations should establish validation processes to evaluate and verify AI outputs. This includes monitoring AI performance, comparing predictions with ground truth data, and regularly reviewing the accuracy and reliability of AI-generated insights. Human oversight can identify any anomalies, validate models against real-world scenarios, and make necessary adjustments to enhance accuracy and ensure the AI system aligns with business goals.
- Exception Handling and Adaptability: AI systems may struggle to handle unique situations and unforeseen events. Organizations should define protocols for handling exceptions and outliers that fall outside the scope of AI algorithms’ predictions. Human experts should be involved in monitoring for situational changes, making timely adjustments to inventory levels, and ensuring responsiveness to unpredictable market dynamics.
- Continuous Learning and Improvement: AI algorithms can adapt to changing patterns, but they require regular updates and improvements to remain effective. Organizations should continuously monitor and assess the performance of AI models, leveraging human insights to identify areas for refinement and enhancing the AI system’s capabilities. By combining the power of AI and human insights, organizations can continuously refine their inventory management strategies and respond effectively to evolving market demands.
- Collaborative Decision-Making: Foster collaboration between AI experts, data scientists, and inventory management professionals. By integrating diverse perspectives, organizations can achieve a comprehensive and well-rounded approach to decision-making. This collaborative environment cultivates continuous learning and knowledge sharing, allowing for more effective and ethical use of AI in inventory management.
By maintaining a balance between AI capabilities and human judgment, organizations can achieve better risk management, maintain control over their inventory management strategies, and ensure that AI-driven decisions align with broader business goals and strategies. The combination of AI and human expertise will contribute to more nuanced decision-making, improved responsiveness to changing market dynamics, and ultimately, optimized inventory control.
To effectively address the risks and challenges associated with AI in inventory management, organizations can implement the following strategies:
- Data Quality Assurance:
Establish data quality control processes to ensure accurate, complete, and reliable data. Regularly audit and clean data to minimize errors and discrepancies that can negatively impact AI-driven inventory management decisions. Implement data governance practices, such as standardized data entry protocols and data validation checks, to maintain data integrity. - Robust Data Integration:
Invest in data integration to consolidate data from various sources and systems within the organization. This ensures a unified view of inventory across the supply chain, facilitating accurate demand forecasting and inventory optimization. Establish data mapping frameworks and data integration platforms to streamline data flow and maintain data consistency. - Scalable Infrastructure:
Adopt scalable AI infrastructure that can accommodate the growing volume of data and evolving business needs. This ensures that AI systems can continue to generate accurate and timely insights as the organization expands. Cloud-based solutions can provide flexibility and scalability, enabling efficient data processing and analysis without extensive upfront infrastructure costs. - Skill and Knowledge Development:
Invest in training and upskilling employees to understand AI concepts, interpret AI-generated insights, and effectively leverage AI for inventory management decisions. Collaborate with data scientists, AI experts, and inventory management professionals to bridge the gap between technical AI knowledge and operational expertise. Foster a culture of continuous learning and knowledge sharing within the organization. - Continuous Monitoring and Improvement:
Regularly monitor the performance of AI systems, evaluate key inventory metrics, and gather feedback from inventory managers and frontline employees. Assess the accuracy and reliability of AI-generated insights against real-world outcomes and make necessary adjustments to optimize inventory management processes. Continually evaluate data quality and the performance of AI models to ensure ongoing improvements and effectiveness.
By proactively implementing these strategies, organizations can mitigate risks and overcome challenges associated with AI in inventory management. They can leverage AI’s capabilities to achieve operational excellence, improve customer satisfaction, and gain a competitive advantage in the market. Continuous monitoring and improvement will ensure that the AI systems remain effective and aligned with evolving business needs and objectives.
Conclusion:
In conclusion, the integration of Artificial Intelligence (AI) into inventory management has the potential to revolutionize supply chain operations and drive significant business growth. AI tools offer numerous benefits, including accurate demand forecasting, process automation, and optimal inventory control. By leveraging these capabilities, organizations can achieve remarkable efficiency gains, cost savings, and improved customer satisfaction.
However, it is important to acknowledge and address the risks and challenges that come with AI implementation. Data quality assurance is crucial to ensure accurate and reliable insights. Organizations must establish data quality control processes and invest in data integration capabilities to ensure the seamless flow of data across different platforms and systems. Moreover, implementation costs can be a barrier, particularly for smaller businesses. A thorough cost-benefit analysis is essential to justify the investment and identify the areas where AI will have the most substantial impact.
Additionally, overreliance on AI can pose risks. While AI algorithms demonstrate high accuracy, they may not account for sudden shifts in customer behavior, market dynamics, or unforeseen external events. Striking the right balance between AI capabilities and human judgment is crucial. Human oversight and expertise are necessary to validate AI outputs, make exceptions for unique situations, and ensure that AI-driven decisions align with business goals and strategies.
To successfully implement AI in inventory management, organizations should follow a well-formed plan. This includes implementing strategies for data quality assurance, robust data integration, scalable infrastructure, skill development, and continuous monitoring and improvement. By proactively addressing risks, leveraging AI tools, and emphasizing collaboration between teams, businesses can unlock the full potential of AI in inventory management.
The time to embrace AI in inventory management is now. Organizations that leverage AI technologies will gain a competitive advantage in today’s fast-paced and data-driven business landscape. By harnessing the power of AI for demand forecasting, process automation, and optimal inventory control, companies can achieve operational excellence, improve customer satisfaction, and ultimately drive business success in an ever-evolving market.